FakET: new method advances electron microscopy with AI simulations
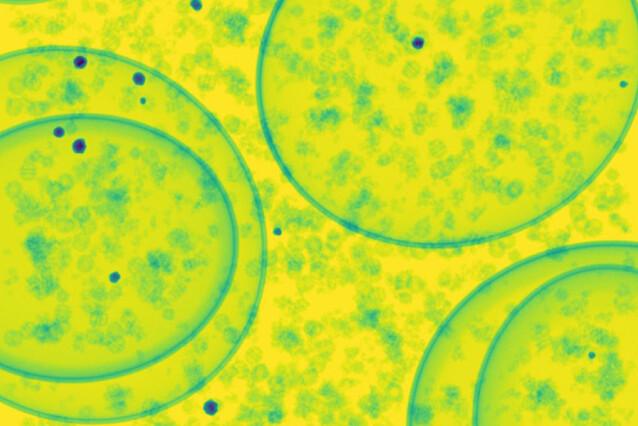
Cryogenic Electron Microscopy (cryo-EM) enables structural biologists to capture high-resolution images, offering a close-up view of biological processes at the near-atomic level. Despite its breakthroughs, challenges such as a limited viewing angle, radiation damage, and shot noise complicate the identification of microscopic particles within these images. To tackle these obstacles, the Cryo-EM Technology Platform at the IMP in collaboration with Pavol Harar at the University of Vienna, developed ‘FakET’. This new data-driven method efficiently generates ‘fake’ electron microscopy images to train Artificial Intelligence analysis software, minimising labour-intensive workflows in particle identification. Their findings are now published in the journal Structure.
Through cryogenic electron microscopy (cryo-EM), structural biologists obtain high-resolution images that allow them to observe biological processes at near-atomic level. Scientists first flash-freeze the samples in a thin film of ice, then use a beam of electrons to capture multiple 2D images. These images are then combined by computer algorithms to create precise 3D models of proteins, viruses, and structures down to the atomic level in some extreme cases.
Within cryo-EM, cryogenic Electron Tomography (cryo-ET) is a specialised application. It involves capturing multiple 2D images of the same sample from various angles and then combining them using advanced algorithms to form a tomogram—a detailed 3D model. Unlike cryo-EM's focus on individual molecules, cryo-ET provides a broader view of biological structures in their natural environments, capturing their spatial context and interactions within the cell. After reconstructing a tomogram, scientists analyse the 3D volume to identify and classify microscopic particles. This is essential for accurately interpreting the biological functions and interactions of structures in the complex and densely packed cellular environment.
Despite recent advancements, several limitations hinder the streamlining of this process. Equipment constraints restrict imaging to a 140 degree range, resulting in a ‘missing wedge’ of data in the final 3D reconstruction. Additionally, the high-energy electron beam used in cryo-EM damages biological samples, requiring a low electron dose to minimise harm, which leads to noisier images. Identifying and analysing molecules within these noisy reconstructions is challenging. To derive high-resolution structures from noisy images, multiple such images are averaged and thereby de-noised.
To support this process, researchers are turning to machine learning algorithms, a subfield of Artificial Intelligence (AI). These sophisticated programs can be trained to efficiently identify particles within cryo-ET images. To achieve desired accuracy, this requires extensive training data with precise annotations, also known as the ‘ground truth’ in the machine learning field. However, the structural biology field is currently lacking readily available and well-annotated data to develop and test such software.
David Haselbach, Technology Platform Head for cryo-EM at the IMP, together with Pavol Harar, Machine Learning Research Engineer at the University of Vienna from Philipp Grohs’ group, have now developed ‘FakET’. This multidisciplinary effort brought together Haselbach’s expertise in cryo-EM and Harar’s skills in data science and machine learning. This new data-driven method can create realistic, computer-generated images that mimic those produced by real electron microscopes, helping scientists train their analysis software in a much less labour intense workflow. Their findings are now published in the journal Structure.
AI simulations boost molecule identification
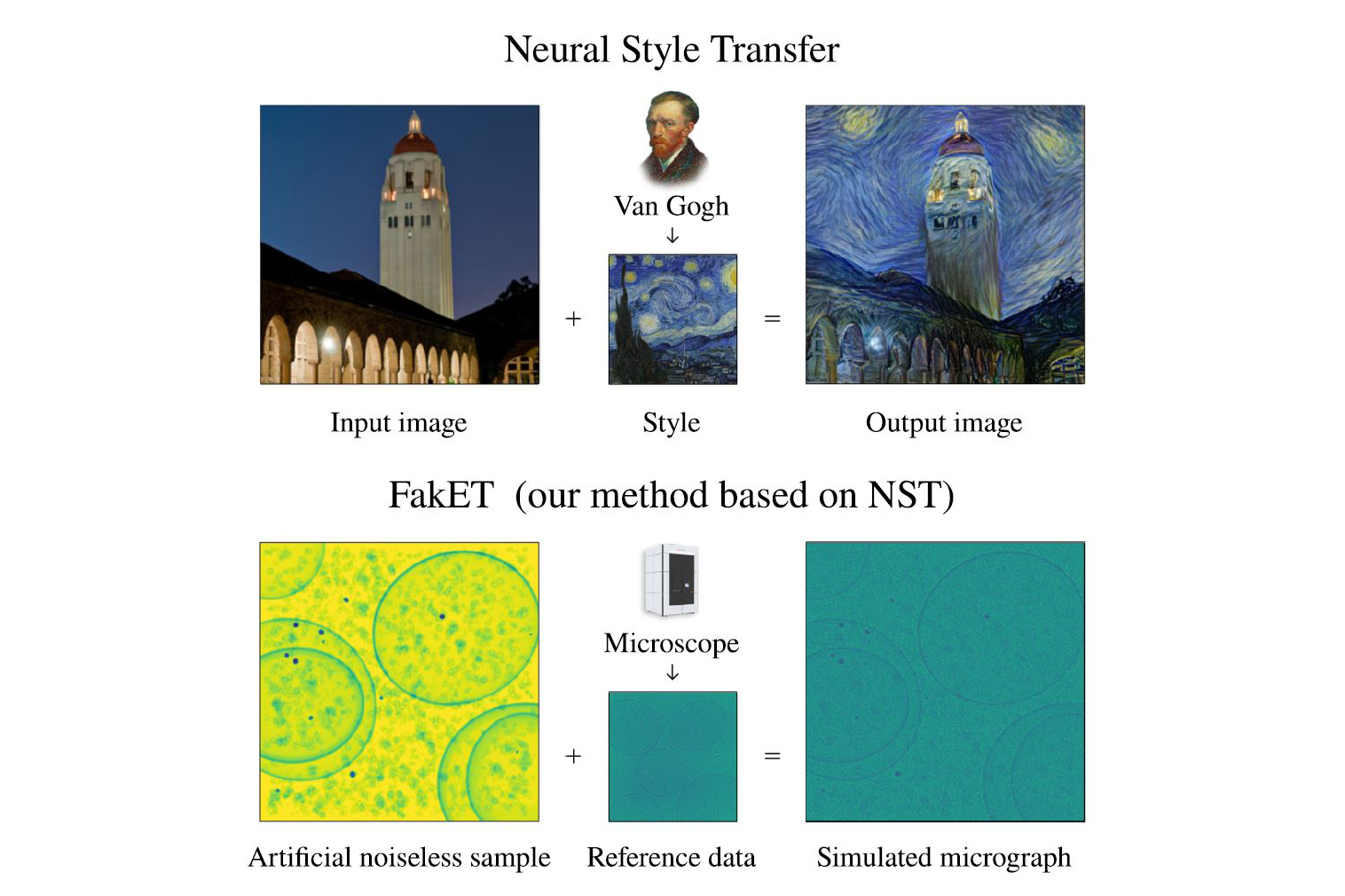
In the fields of structural biology and visual proteomics, identification of molecules in cellular tomograms is a task of great importance. Scientists nowadays employ AI tools to significantly streamline this process, however scarcity of properly annotated training tomographic images hinders this progress. To address this challenge, researchers often rely on simulators to generate synthetic tomograms with known annotations.
“Physics-based simulators that mimic the creation of tomograms are available but are often impractical due to their high computational demands,” says David Haselbach. “This prompted us to investigate if machine learning could help generate these tomograms faster and more efficiently.” This led to the development of FakET, a machine learning tool capable of generating images that closely resemble those captured by electron microscopes.
These realistic images are crucial for teaching computers how to identify different particles. “It’s like creating a virtual zoo filled with accurately labelled animals to teach a computer how to recognise each species in the wild,” says Pavol Harar, first author of the study, and currently Computational Scientist at the Institute of Science and Technology Austria (ISTA). “FakET uses neural style transfer, a technique that employs pretrained neural network to mix content and style of two images. It applies the artistic style of a reference image, such as a painting, onto another image depicting the desired content, such as a photograph. This process generates a new composite image, merging the desired content of one image with the artistic style of the reference.”
We use a YouTube plugin to display social media content on this page, which places requests to YouTube servers. These requests make your IP address visible to YouTube, who may use it in accordance with their data privacy policy. Please agree to make the YouTube video visible. You can find more information in our privacy settings.
AcceptIn this case, FakET learns the unique ‘style’ of the noise that the microscope naturally adds to its images. This makes the simulated pictures very realistic, almost as if taken directly from the microscope. It is designed to be easy—scientists can simply input in their desired ‘protein soup’ and a reference image from the microscope, and FakET then creates high-quality training data for teaching computers how to identify and classify particles.
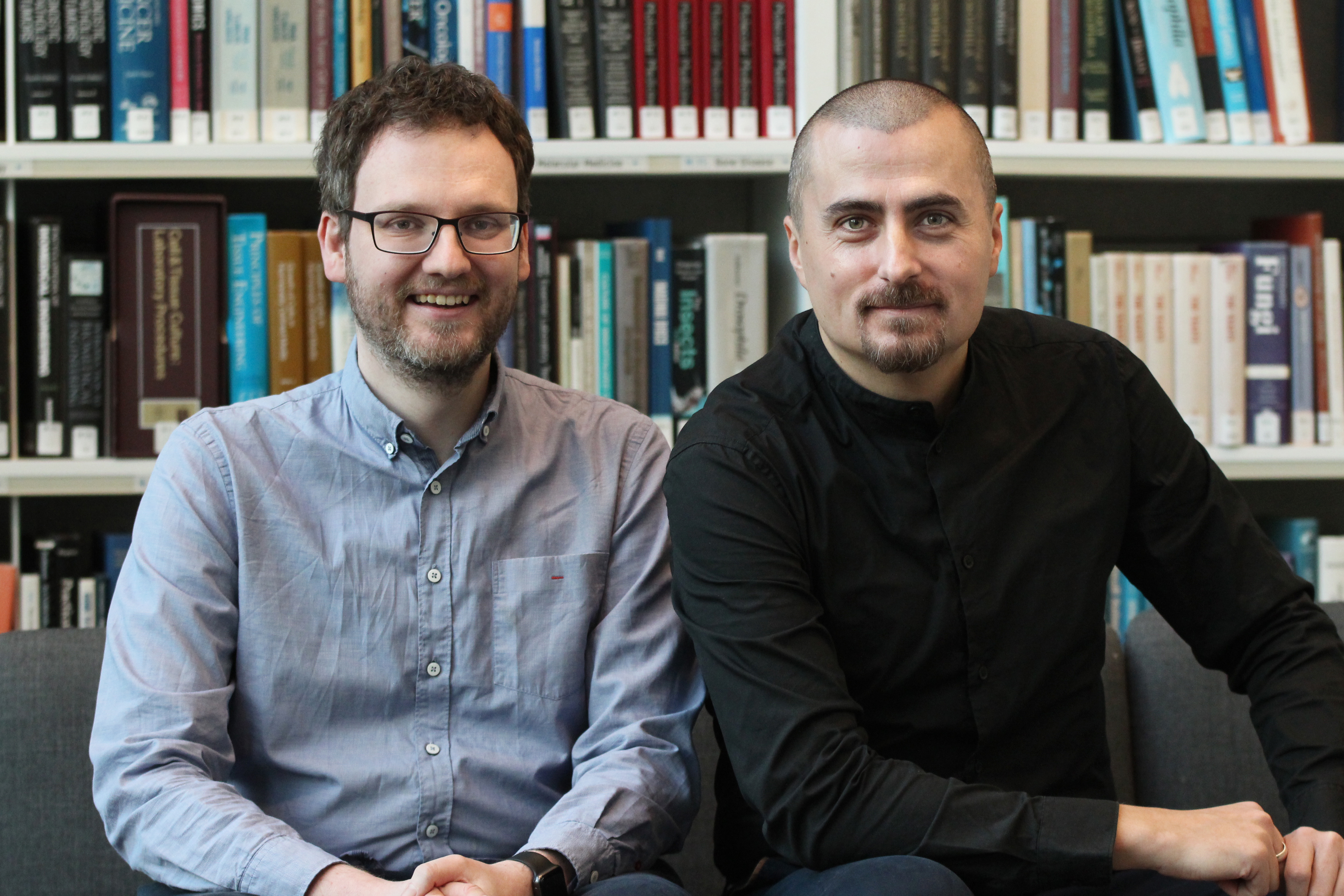
“This project has been a multidisciplinary collaboration,” says Haselbach. “I brought the challenge from structural biology, while Pavol brought his expertise in machine learning. Together, we developed a tool that not only simplifies image analysis but has the potential to advance cryo-ET imaging capabilities by streamlining it further.”
With a pre-trained model ready to use, researchers can quickly and efficiently produce synthetic images from any cryo Electron Microscope they have a reference image from. “Our approach is innovative in replacing the time-consuming and laborious task of manual data annotation,” says Haselbach. “We think it will pave the way for new applications based on deep learning that can further streamline cryo-ET image analysis.”
Original Publication
Pavol Harar#, Lukas Herrmann, Philipp Grohs, and David Haselbach#. "FakET: Simulating Cryo-Electron Tomograms with Neural Style Transfer." Structure, DOI: 10.1016/j.str.2025.01.020
#Corresponding authors.
About the IMP at the Vienna BioCenter
The Research Institute of Molecular Pathology (IMP) in Vienna is a basic life science research institute largely sponsored by Boehringer Ingelheim. With over 220 scientists from 40 countries, the IMP is committed to scientific discovery of fundamental molecular and cellular mechanisms underlying complex biological phenomena. The IMP is part of the Vienna BioCenter, one of Europe’s most dynamic life science hubs with 2,800 people from over 80 countries in six research institutions, two universities, and 35 biotech companies. www.imp.ac.at, www.viennabiocenter.org
Further reading