Harnessing artificial intelligence to predict and control gene regulation
The human genome contains hundreds of thousands of enhancer sequences that switch our genes on and off when needed. Scientists have long tried to decipher the link between the enhancer sequence and its regulatory activity in the cell, with little success. The lab of Alexander Stark at the IMP has developed a deep learning model, DeepSTARR, which predicts enhancer activity from their DNA sequence with exceptional accuracy. The scientists extracted the rules learned by the model and used them to design synthetic enhancers with a desired level of activity. Their work is now published in the journal Nature Genetics.
Our genes provide the instructions to produce all the proteins that our bodies need to function. While all tissues in one body harbour the same genes, they do not use (or ‘express’) them uniformly. Genes in different tissues are activated on-demand thanks to regulatory DNA sequences called ‘enhancers’, which act as on-off switches on gene transcription.
The tissue-specific switching of genes is encoded in DNA’s famous four-letter alphabet, A, T, G, and C. Some hundred DNA letters specify the activation of a gene in a cell, in a coded language that scientists have struggled to decrypt. For decades, geneticists have tried to elucidate the rules that determine the connection between an enhancer’s sequence of DNA bases and its regulatory activity in the genome. Understanding how a sequence influences gene expression could allow researchers to create synthetic enhancers from scratch and control the expression of a gene of interest.
Scientists in the lab of Alexander Stark at the IMP have risen to the challenge and utilised artificial intelligence for the task. They developed a powerful deep learning model, called DeepSTARR, which can predict the activities of any enhancer sequence, and validated their model experimentally. The outcome was now published in the journal Nature Genetics.
Taming the power of deep learning
Enhancers are made of a series of ‘motifs’ that are bound by specific types of proteins called transcription factors to activate gene transcription. Determining how each enhancer’s sequence and motifs encode its activity across an entire genome would be a herculean task, were it to be done manually. Instead, the Stark Lab has harnessed the potential of deep learning to unveil the rules that govern enhancer activity.
Deep learning models are a powerful technology suited to process large, raw datasets, and to identify the rules that optimise an output specified by the programmer. The researchers applied such a model to the genome of a type of fruit fly cell: they successfully identified enhancers and predicted enhancer activity from the arrangement of their As, Ts, Gs, and Cs.
“Motifs are a bit like words arranged in a particular order. By themselves, words give us some information about their meaning, but that meaning can change in the context of a sentence. Similarly, motifs need to be analysed within their context to understand how they orchestrate enhancer activity,” explains Bernardo de Almeida, Vienna BioCenter PhD student and first author of the study. “Our model was able to learn the ‘syntax’ rules of enhancer motifs to predict enhancer activity very accurately.”
“We expanded and validated our findings to find universal rules that would apply to other cell types, and to other species, including humans,” says Franziska Reiter, also Vienna BioCenter PhD student in the lab of Alex Stark. “There are few labs in the world that can develop powerful computational tools and directly validate them with real life experiments. Our lab is one of them.”
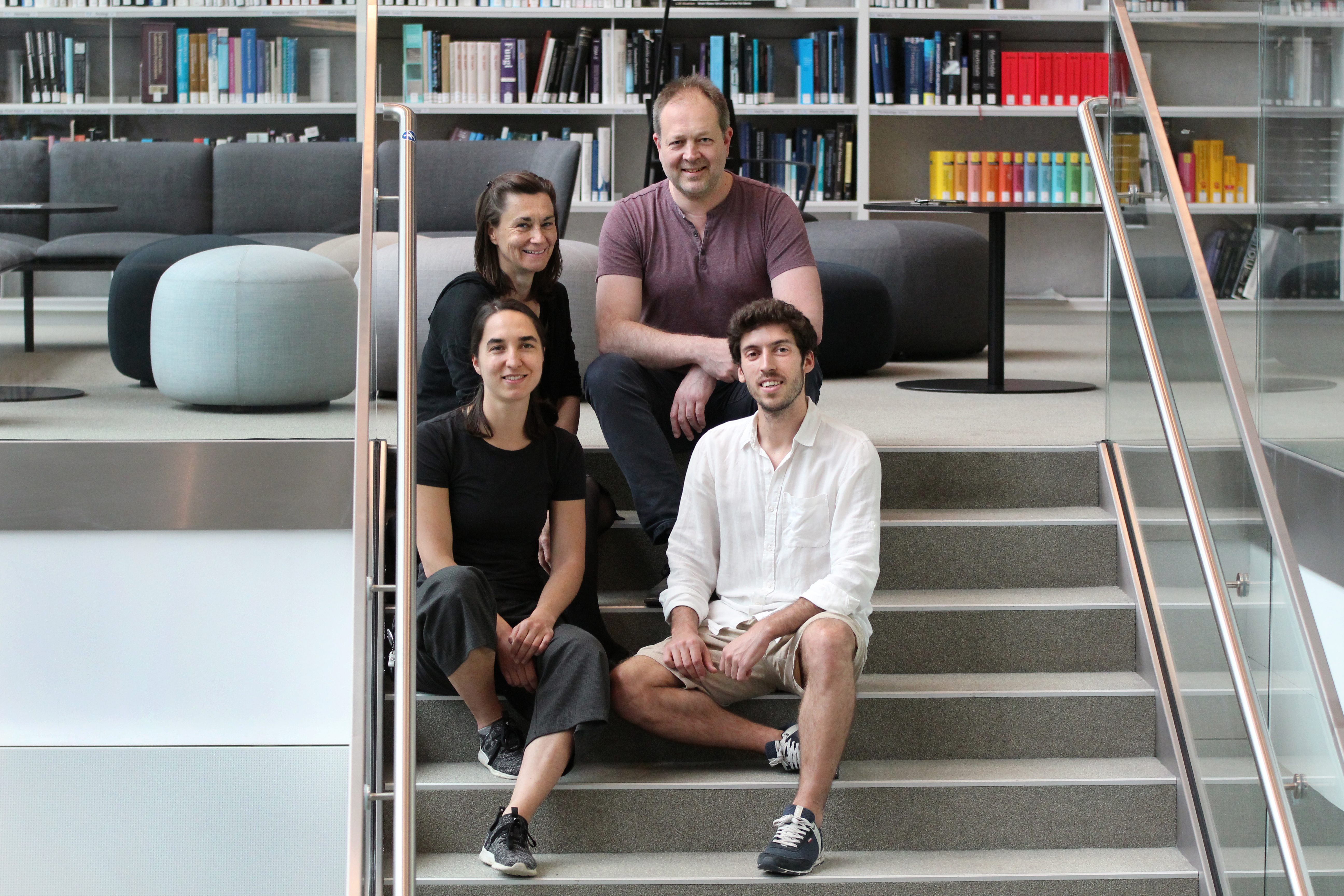
You can build, so you understand
To test the power of DeepSTARR, the scientists used its predictions to design and generate new enhancer sequences in fruit fly cells. They were able to customise the strength of the synthetic enhancer’s activity by following the rules that DeepSTARR had pinpointed. This shows that the model was able to learn the correct syntax rules of the enhancers’ mysterious ‘language’.
“The engineering of synthetic enhancers with desired properties provides unanticipated opportunities for controlling gene expression, with future applications for cell and gene therapy,” says de Almeida. “Our work shows the potential of deep learning models to learn the codes that rule the natural world at the smallest scales.”
“DeepSTARR achieves what I had come to do at the IMP since 2008. Our study demonstrates the power of combining computational biology and experimental work – an approach that sits at the heart of my lab’s philosophy," says Alex Stark. “Today is a very special day for me. I couldn’t be prouder of my lab for their creativity, hard work, and dedication.”
Original publication
Bernardo P. de Almeida, Franziska Reiter, Michaela Pagani, Alexander Stark: "DeepSTARR predicts enhancer activity from DNA sequence and enables the de novo design of synthetic enhancers". Nature Genetics (2022). DOI: 10.1038/s41588-022-01048-5.
Further reading